By Massimo Petrini, Daniela Pellegrino, Emiliano Casale, TERNA RETE Italia, Italy
In the complex landscape of electrical power systems, the pivotal role played by PAC systems cannot be understated. These systems form the bedrock for an efficient power generation, transmission, and distribution, providing a sophisticated network of controls and safeguards to ensure seamless operation and protection against potential faults.
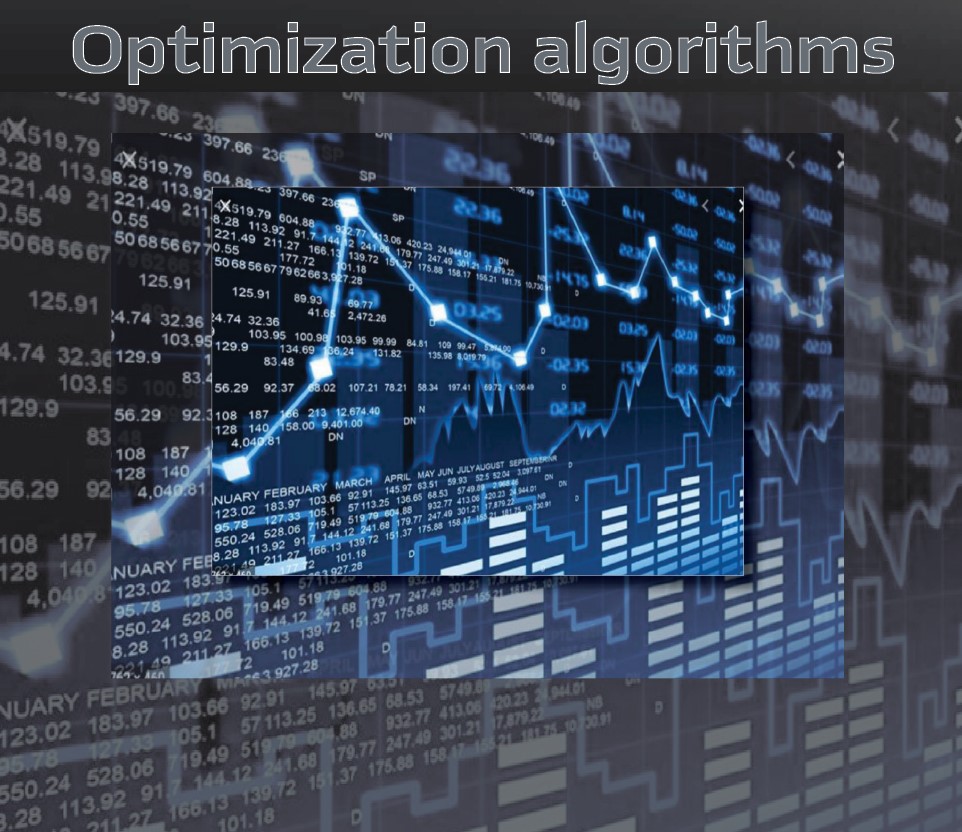
The reliability and effectiveness of PAC systems directly impact the overall performance and resilience of the electrical grids.
The traditional paradigms of PAC systems have excelled in providing essential functionalities, such as fault detection and protection mechanisms, ensuring the stable and secure functioning of power networks. However, the increasing complexity of modern power systems, coupled with the evolving nature of potential challenges, requires a paradigm shift in the way these systems operate and respond to dynamic conditions.
The integration of Artificial Intelligence (AI) technologies heralds a new era in the evolution of PAC systems. AI introduces a layer of intelligent decision-making, adaptability, and automation that transcends the capabilities of conventional systems. By leveraging AI, PAC systems have the potential not only to enhance their existing functionalities, but also to usher in a range of novel features that address the complexity of the contemporary power grid challenges.
The promise of AI lies in its ability to analyze vast amounts of data in real-time, identify patterns, and make informed decisions autonomously. This transformative capability is particularly relevant in the context of PAC systems, where rapid response to faults, adaptive protection settings, and predictive maintenance are critical for ensuring the reliability and resilience of the power infrastructure.
Moreover, the integration of AI in PAC systems aligns them with the broader industry trends of digitalization and the Internet of Things (IoT). It represents a strategic response to the growing need for intelligent, data-driven solutions that can optimize power system performance, mitigate risks, and provide a foundation for future innovations in the energy sector.
This paper delves into the profound impact of AI on PAC systems, exploring its applications in fault detection, adaptive protection settings, asset management, dynamic line rating, grid optimization, image analysis, and the integration of Virtual Reality (VR) and Augmented Reality (AR) technologies. By examining these facets, we aim to illuminate the extensive capabilities that AI infusion brings to PAC systems, ultimately contributing to a more intelligent, adaptable, and automated power grid.
Dynamic Line Rating and Grid Management Optimization
The integration of Artificial Intelligence (AI) into Dynamic Line Rating (DLR) and Grid Management Optimization within the domain of Protection, Automation, and Control (PAC) systems revolutionizes the management of power grids, ushering in an era of unprecedented efficiency and reliability. By harnessing AI, particularly in predicting real-time temperature conditions, these systems gain the capability to dynamically adjust line ratings, optimize electricity transmission, and prevent overloads. Additionally, AI facilitates accurate forecasting of electricity demand, thereby enhancing grid operations and supporting the seamless integration of renewable energy sources.
AI’s ability to predict real-time temperature conditions is instrumental in enhancing the accuracy of Dynamic Line Rating systems. Traditionally, power lines are rated based on conservative assumptions about environmental conditions. However, AI leverages real-time data on weather conditions, solar radiation, wind speed, and other relevant factors to predict the current temperature along the power lines. This dynamic approach enables a more precise assessment of the thermal capacity of transmission lines in real-time.
By continuously updating temperature predictions, AI-equipped systems can swiftly respond to changing weather patterns and environmental conditions. This dynamic adjustment ensures that line ratings accurately reflect the current thermal capacity, optimizing transmission efficiency and preventing the risk of overloads that may lead to equipment failures or service interruptions.
The dynamic adjustment of line ratings facilitated by AI leads to the optimization of electricity transmission. As environmental conditions change, AI algorithms continuously assess the thermal constraints of power lines and adjust their ratings accordingly. This ensures that the power lines operate at their maximum allowable capacity while maintaining safety margins.
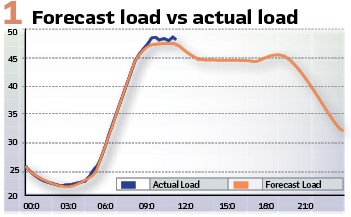
Optimizing electricity transmission not only improves the overall efficiency of the grid, but also allows the utilization of existing infrastructure to its full potential. This is particularly crucial in scenarios where increased demand or the integration of renewable energy sources requires a more robust and flexible power transmission network. (Figure 1).
Another benefit of AI-driven Dynamic Line Rating is the ability to prevent overloads. By accurately predicting real-time temperature conditions and adjusting line ratings accordingly, the system can proactively identify potential overloads before they occur. This preventive measure mitigates the risk of equipment damage, reduces the likelihood of unplanned outages, and enhances the overall reliability of the power grid.
AI’s application in forecasting electricity demand is a key element in optimizing grid operations. Machine learning models analyze historical consumption patterns, consider economic indicators, and incorporate real-time data to predict future electricity demand accurately. This forecasting capability empowers grid operators to anticipate peak demand periods, plan for efficient energy generation and distribution, and optimize grid resources accordingly.
Attention must also be paid to the variability and intermittency of renewable energy sources which pose challenges to grid stability. AI plays a pivotal role in addressing these challenges by forecasting the generation patterns of solar and wind power. By understanding the expected output from renewable sources, grid operators can proactively adjust grid parameters, storage systems and demand response mechanisms to accommodate fluctuations in renewable energy production. AI-driven optimization strategies allow for the effective integration of renewable energy into the grid, maximizing the utilization of clean energy sources while maintaining grid stability. This contributes to the overall sustainability of the power grid, aiding the global efforts to transition towards a more environmentally friendly and resilient energy infrastructure.
In summary, the integration of AI in Dynamic Line Rating and Grid Management Optimization brings about a paradigm shift in power grid operations. By predicting real-time temperature conditions, dynamically adjusting line ratings, optimizing electricity transmission, and forecasting electricity demand, AI empowers utilities to operate grids more efficiently, prevent overloads, and seamlessly integrate renewable energy sources, ultimately enhancing the reliability, sustainability, and resilience of the power infrastructure.
Adaptive Protection Systems
The integration of Artificial Intelligence (AI) into Adaptive Protection Systems within the domain of Protection, Automation, and Control (PAC) marks a transformative leap forward in the orchestration of power grid operations. By harnessing AI, particularly through AI-driven load forecasting models and Dynamic Line Rating, indicated in the previous section, these systems gain the proactive adaptability needed to respond effectively to changing load conditions, thereby optimizing protection settings and fortifying the efficiency and reliability of the power grid.
Traditional protection systems often operate based on fixed settings, which lack the agility required to accommodate dynamic changes in the load profile. In contrast, AI-driven load forecasting models play a pivotal role in enabling Adaptive Protection Systems to anticipate and respond to fluctuations in load demand.
By employing sophisticated machine learning algorithms, AI can be trained on historical data to discern complex patterns and trends, enabling precise predictions regarding future load variations. This foresight empowers the Adaptive Protection System to preemptively adjust protection settings, ensuring optimal performance even in the face of unforeseen load fluctuations. For example, AI algorithms can dynamically tweak protection settings based on the current state of the power system, optimizing them during periods of high demand or network reconfiguration in order to prevent unnecessary tripping.
Dynamic Parameter Adjustment serves as a cornerstone in the adaptability of Protection Systems, guided by insights gleaned from AI-driven forecasting models. Continuous monitoring of critical variables such as load demand, voltage levels, and frequency deviations is central to the Dynamic Parameter Adjustment process. As deviations from normal operating conditions are detected, protection settings are dynamically modified to meet the requirements of the current system state. This dynamic adaptability ensures that the protection system remains responsive and effective, mitigating potential risks associated with transient grid variations.
The core objective of Dynamic Parameter Adjustment is to optimize protection settings in real-time. By leveraging historical data patterns and AI-driven insights, the protection system can fine-tune parameters such as trip settings, time delays, and coordination schemes. This optimization not only facilitates the rapid identification and isolation of faults, but also minimizes the likelihood of false trips during normal grid operations.
Furthermore, the ability to adapt protection settings based on load forecasting enables the system to preemptively address potential challenges associated with varying load demand. This proactive approach not only enhances the stability of the power grid, but also contributes to a reduction in downtime, thereby optimizing the overall operational efficiency of the electrical infrastructure.
In conclusion, the integration of AI into Adaptive Protection Systems empowers these systems with the foresight and adaptability needed to navigate the complexities of modern power grids.
AI in Fault Detection and Classification
The incorporation of Artificial Intelligence (AI) into Fault Detection and Classification within Protection, Automation, and Control (PAC) systems represents a transformative leap in the realm of power grid management. By harnessing the capabilities of AI, particularly through real-time data analysis and advanced machine learning models, PAC systems can now respond with unprecedented speed and accuracy to faults, ushering in a new era of proactive fault management.
In the context of fault detection and classification, AI revolutionizes the response of electrical systems. For instance, in pattern recognition, machine learning models are trained on historical data to recognize patterns associated with different fault types, enabling real-time identification. AI-driven data analytics processes large volumes of data in real-time, identifying deviations and triggering alerts or predefined actions based on fault patterns. (Figure 2).
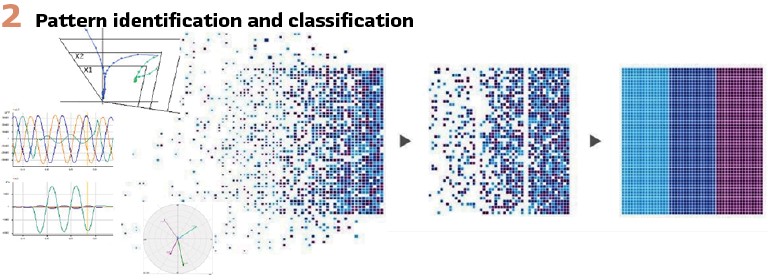
Deep learning techniques, such as neural networks, serve as powerful tools for fault detection and classification. These models autonomously learn and adapt to complex patterns in data, thereby improving the accuracy of fault identification.
Feature extraction is another capability of AI algorithms, automatically extracting relevant features from raw data to focus on key fault indicators, reducing analysis complexity, and enhancing efficiency.
Ensemble learning techniques involve combining multiple AI models to improve fault detection accuracy by leveraging the strengths of different algorithms, providing a more robust and reliable fault classification. In cases with limited labeled fault data, unsupervised learning techniques come into play, allowing AI algorithms to identify anomalies without prior knowledge of fault patterns, making them adaptable to evolving system conditions.
Real-time monitoring facilitated by AI ensures continuous observation of the power system. Upon detecting a fault, the system can take immediate actions, such as isolating the faulty section or reconfiguring the network, to minimize the impact. Adaptive systems in AI-based fault detection enable the algorithms to evolve with changes in the power system over time. As the system undergoes transformations or new fault types emerge, the AI algorithms can be updated and retrained to maintain accurate fault detection and classification capabilities.
Integration with SCADA systems further enhances fault detection by combining AI’s analytical capabilities with real-time SCADA data, providing a comprehensive approach to fault identification and response. By leveraging AI in fault detection and classification, electrical systems can significantly improve responsiveness, reduce downtime, and enhance overall reliability by swiftly identifying and addressing faults in the power grid.
In summary, the infusion of AI into Fault Detection and Classification in PAC systems empowers the grid with a level of responsiveness and accuracy that was previously unattainable. Through continuous real-time analysis and the application of advanced machine learning models, AI not only detects faults swiftly but also lays the foundation for intelligent fault severity assessment, ushering in a paradigm shift towards proactive fault management in power grid operations.
Asset Management and Predictive Maintenance
The infusion of Artificial Intelligence (AI) into Asset Management and Predictive Maintenance within the ambit of Protection, Automation, and Control (PAC) systems heralds a transformative approach to ensure the longevity and performance of critical grid components. AI algorithms, when applied to assess the health of grid assets, leverage a multifaceted approach, encompassing historical performance analysis, consideration of environmental conditions and real-time operational data. This comprehensive strategy facilitates a paradigm shift from reactive to proactive maintenance, empowering utilities to make informed decisions and promptly respond to potential disruptions.
AI algorithms delve into the historical performance data of grid assets to discern patterns, anomalies, and potential indicators of degradation or impending failures. By analyzing trends over time, these algorithms can identify subtle changes in asset behavior that may serve as early warning signs of deterioration. This historical perspective enables utilities to understand the asset’s performance baseline, track its evolution, and anticipate potential issues before they escalate into critical failures.
In addition to historical performance analysis, AI algorithms consider environmental conditions and real-time operational data to gain a holistic view of the factors influencing asset health. Environmental factors such as temperature, humidity, and pollution levels can significantly impact the wear and tear of grid components. By incorporating this information into the analysis, AI algorithms can discern correlations between environmental conditions and asset degradation, enhancing the accuracy of predictive maintenance models.
Operational data, acquired in real-time, provides crucial insights into the current state of grid assets. Continuous monitoring allows AI algorithms to detect sudden changes or anomalies that may indicate emerging issues. For example, variations in voltage, current, or temperature beyond established thresholds can trigger alerts, prompting proactive intervention before a potential disruption occurs.
The predictive capabilities of AI-driven Asset Management and Predictive Maintenance extend beyond identifying potential issues to forecasting when these issues are likely to manifest. Machine learning algorithms excel at recognizing complex patterns within diverse datasets, enabling them to predict the remaining useful life of assets and anticipate the timing of maintenance interventions.
This predictive foresight empowers utilities to adopt a proactive approach to asset management. Rather than relying on field maintenance schedules, AI algorithms dynamically adjust maintenance plans based on the real-time health status of assets. Predictive maintenance strategies enable utilities to optimize resource allocation, prioritize critical assets, and minimize downtime by addressing issues before they escalate.
The integration of AI in Asset Management facilitates timely responses to potential disruptions by providing utilities with actionable insights. AI algorithms generate alerts and recommendations based on the analysis of historical, environmental, and operational data. These insights guide utilities in making informed decisions regarding maintenance interventions, replacement schedules, or upgrades.
By addressing issues before they escalate, utilities can significantly reduce the risk of unexpected outages, extend the lifespan of assets, and enhance the overall reliability of the power grid. Timely responses enabled by AI-driven predictive maintenance contribute to a more resilient and robust electrical infrastructure.
In conclusion, the adoption of AI in Asset Management and Predictive Maintenance represents a proactive shift towards ensuring the health and longevity of critical grid assets. By leveraging historical performance analysis, considering environmental conditions, and incorporating real-time operational data, AI algorithms empower utilities with predictive capabilities that enable proactive decision-making and timely responses to potential disruptions, ultimately enhancing the reliability and efficiency of power grid operations.
Image Analysis
The application of image analysis in the realm of Protection, Automation, and Control (PAC) systems marks a significant stride toward enhancing the visual intelligence of power grid infrastructure. Image processing algorithms are deployed with precision for the detection of defects and anomalies in crucial components such as disconnectors and switches. By harnessing the power of artificial intelligence, these algorithms can meticulously scrutinize images, identifying imperfections such as cracks, corrosion, loose connections, corona discharges, arcing, or any irregularities that may compromise the performance of these critical elements. (Figure 3).
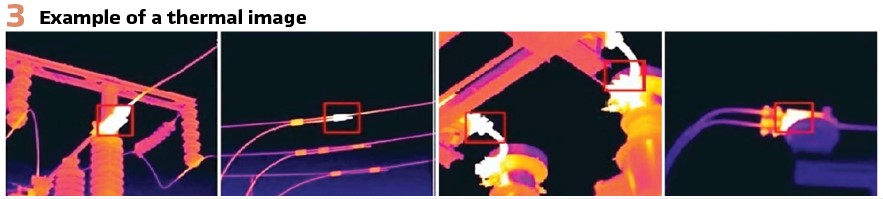
A particularly powerful tool within image analysis is thermal imaging, which extends the capabilities of defect detection to include the identification of hot spots. Thermal imaging enables the visualization of temperature variations, allowing the swift identification of areas experiencing abnormal heat levels. This capability is invaluable for detecting potential electrical faults, identifying components under excessive stress, and preventing catastrophic failures that could result from overheating.
Furthermore, image analysis contributes significantly to remote monitoring and proactive maintenance strategies within the power grid infrastructure. Through the deployment of cameras or sensors equipped with image analysis algorithms, a remote monitoring system can continuously capture visual data from various components. The automatic analysis of these images facilitates the identification of changes in appearance or behavior that may indicate potential issues. In a proactive maintenance context, image analysis serves as a critical tool for condition monitoring. By continuously monitoring visual data, the system can detect early signs of wear and tear, degradation, or impending failures. This information enables utilities to schedule maintenance activities in a predictive manner, addressing potential issues before they escalate into critical failures.
This predictive approach not only minimizes downtime, but also optimizes resource allocation by focusing maintenance efforts on components that genuinely require attention. (see Figure 4). The integration of image analysis into PAC systems, therefore, transforms visual data into actionable insights. It empowers utilities with the ability to remotely monitor critical components, proactively address maintenance needs, and ensure the reliability and performance of power grid infrastructure. As technology continues to advance, image analysis will play an increasingly pivotal role in shaping the future of PAC systems, contributing to a more resilient, efficient, and visually informed power grid.
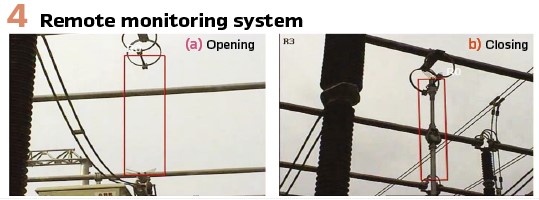
VR and AR Integration
The integration of Virtual Reality (VR) and Augmented Reality (AR) technologies into Protection, Automation, and Control (PAC) systems marks a transformative leap toward revolutionizing equipment monitoring, diagnostics, and maintenance activities within the power grid infrastructure. These immersive technologies enhance the capabilities of technicians by providing them with unprecedented insights and tools for real-time decision-making.
In the realm of equipment monitoring, AR overlays relevant data directly onto physical components. Technicians equipped with AR devices, such as smart glasses or headsets, can access a wealth of information without diverting their attention from the equipment at hand. This includes live data feeds, schematics, manuals, and diagnostic information. Overlaying relevant data in this manner empowers technicians to quickly assess the status of equipment, identify potential issues, and access procedural information or troubleshooting guides, facilitating efficient decision-making and reducing the time required for equipment monitoring.
AR also plays a pivotal role in diagnostics by providing real-time visualizations of system parameters and performance metrics. Technicians can view live data superimposed on physical equipment, enabling them to detect anomalies, analyze trends, and identify potential faults with greater precision. This real-time diagnostic capability allows for swift and accurate identification of issues, leading to more effective troubleshooting and faster resolution of problems within the PAC systems.
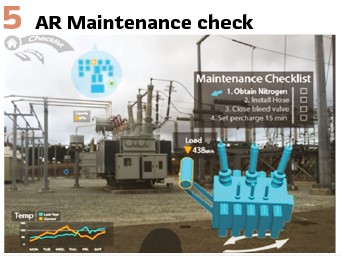
Furthermore, VR and AR technologies bring about immersive visualizations of entire substations, offering a training and monitoring experience that transcends traditional methods. Through VR simulations, operators and technicians can virtually “walk through” substations, inspect equipment, and simulate various scenarios for training purposes. This immersive training approach enhances situational awareness, fosters familiarity with equipment configurations, and allows for realistic simulations of emergency scenarios, contributing to the development of a highly skilled workforce. (Figure 5).
AR overlays on physical equipment also facilitate maintenance activities by providing step-by-step guidance, visual cues, and interactive instructions. Technicians can access real-time information about equipment health, historical maintenance records, and safety guidelines. This augmented guidance ensures that maintenance tasks are performed accurately and efficiently, minimizing the risk of mistakes and enhancing overall maintenance effectiveness.
The benefits of VR and AR integration extend beyond individual tasks, influencing the collaborative aspects of PAC system operations. Remote assistance functionalities, enabled by AR, allow experts to provide guidance to on-site technicians in real time. This not only facilitates efficient problem resolution but also contributes to knowledge transfer and skill development among the workforce.
In conclusion, the integration of VR and AR technologies into PAC systems significantly elevates the efficiency, accuracy, and safety of equipment monitoring, diagnostics, and maintenance activities. By overlaying relevant data on physical equipment, facilitating immersive visualizations for training and monitoring, and enabling real-time collaboration, VR and AR contribute to a more advanced, informed, and connected approach to managing power grid infrastructure. As these technologies continue to evolve, their impact on PAC systems promises to further enhance the capabilities of technicians and operators, ensuring the reliability and resilience of the electrical power grid.
Biographies:

Massimo Petrini received his Degree in Electrical Engineering from the Politecnico di Torino, Italy in 1990. He works for Terna, the Italian TSO, where, since 2022, he is Head of Technology Management Support and has been involved in projects dealing with the integration of the components of the HV power grid. From 1997 to 2022 he led the teams that developed and managed the protection, automation and control systems projects, driving their transition from the electromechanical to the digital technology. He is Cigre B5 member since 2005 and a former B5 Italian Delegate. Massimo has been involved, both as a member and as a convenor, in different Cigre WGs.

Emiliano Casale earned his master’s degree in electrical engineering in 2003. In 2004, he joined Terna, the Italian Transmission System Operator. His expertise spans system integration, standardization, and interoperability using the IEC 61850 model, as well as PACS project development, validation, and training. Currently serving as the Head of Substation Systems, Emiliano is also the Italian National Member of the CIGRE Study Committee B5, focusing on “Protection and Automation.”

Daniela Pellegrino earned her master’s degree in mathematical engineering from the Politecnico di Torino, Italy in 2004. From 2004 to 2006 she developed numerical algorithms in biomedical and industrial fields for a leading consultancy company. From 2006 to 2020 she was a SCADA developer in the PACS and project manager for the development of PACS for Italian and foreign TSOs. Since 2020 at the Polytechnic of Turin, she was a researcher in the fields of VR, AR, AI and computer vision. Since 2021 she joined Terna as SCADA specialist for PACS development and operation.