by Mital Kanabar, GE Grid Solutions, Canada, Sudhanshu Mishra and Balakrishna Pamulaparthy, GE Grid Solutions, India, and Claudia Cosoreanu, Global PM & CTO
Key Drivers in the energy transition and role of AI:
The energy industry is currently undergoing a significant transition from fossil fuels to sustainable energy sources. This shift is driven by the imperatives of decarbonization and electrification, as well as the UN’s Sustainable Development Goals (SDGs) such as, climate action, and initiatives for affordable and clean energy. However, this transition needs to balance the dimensions of the energy trilemma: energy security (including, reliability and resilience), affordability or equity, and environmental sustainability.
The energy transition is imminent, but its success relies on our ability to adapt our aging power grid infrastructure to support the entire ecosystem surrounding it. Innovation and the digital transformation of the grid are pivotal for rapidly evolving various technologies.
One of the key technologies enabling energy transition is, Artificial Intelligence (AI), esp. physics-informed AI, as illustrated in Figure 1. Artificial Intelligence, despite its associated concerns and excitement, holds immense promise. Similar to any other powerful tool, it can be applied in diverse ways. To simplify the concept of AI, let’s draw an analogy to the human brain. Consider the human senses: sight, hearing, touch– and all collecting information, while our limbs act as actuators for responses. This information, or data, is then segregated by the human brain to comprehend our surroundings, experiences, and learnings. Artificial intelligence utilizes these human brain-like functionalities through what we call as “models” – designed to perform data science tasks and specific analysis. These algorithms are programmed to recognize patterns and utilize data to essentially make decisions. In an intelligent machine, AI processing has sensors as inputs and actuators as outputs. In the development of AI, we essentially train and program the machine with various scenarios of inputs and outputs.
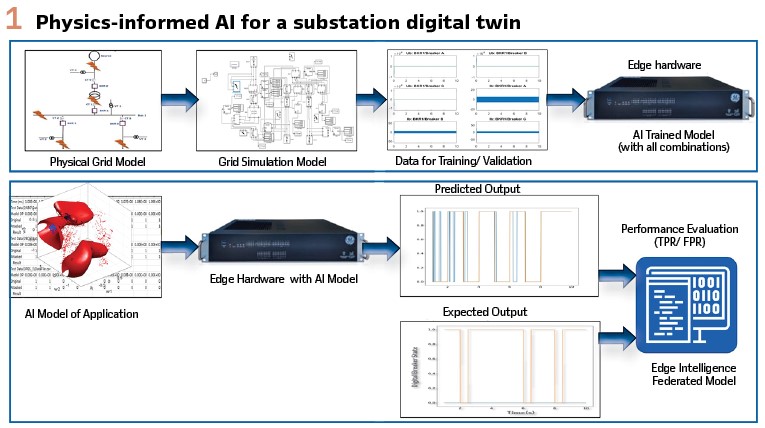
Much like how humans learn to avoid touching hot surfaces through the experience of getting hurt as the outcome, machines acquire learnings based on the inputs and outputs user provide – a process known as model training. Once the training is complete, a test is performed with pass/fail criteria, resulting in predicative algorithms. As machines receives more data, the accuracy of the forecasts and analyses improves.
Terminologies – AI/ML/DL?
There are few terms that are very commonly associated with AI, including Machine Learning (ML), Deep Learning (DL) and more recently discussed like, Generative AI (Gen AI). Overall, they all fall under the broader umbrella of AI, which pertains to how computers or machines can mimic human intelligence. Machine learning (ML) is a subset of AI that focuses on how machines trains on tasks and enhance their decision-making or prediction accuracy through experience.
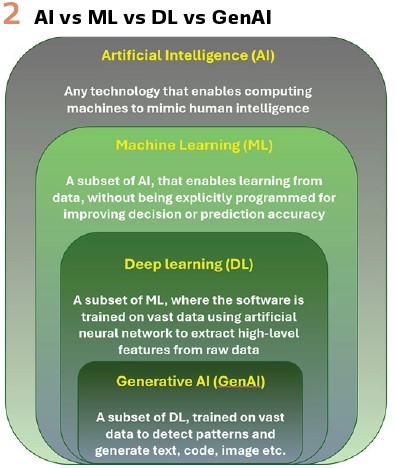
Deep Learning (DL) is a specific subset of ML. In DL, models (software) are trained on vast amounts of data using advanced artificial neural networks structures of, capable of extracting high-level features from raw input data.
Generative AI (GenAI) is a specialized subset of DL. It can generate content such as text, code, images etc. based on provided inputs. GenAI is trained on extensive data that helps models to detect patterns and generate output without explicit programming. Figure 2 illustrates these concepts graphically.
AI – Strengths & Weaknesses
Like any technology, AI possesses strengths and weaknesses. Here are a few of them:
Strengths:
- Automated decision-making: The decision-making process for AI lies in the data on which it is trained. If the data is collected with minimal or no bias, or if bias is removed during preprocessing, then we are certain that the AI will provide unbiased output. In contrast, when there is human involvement in direct data analytics or explicit programming, there are high chances that the decisions are biased based on prior experiences such as., tasks involving filtering job applications, or making promotion decisions.
- Pattern identification: Despite advances in research and development in finding multiple insights to traditional complex problems, the capability of extraction of insights by AI is still unmatched. The traditional grid was well defined by the laws of physics and any transient behavior could be modeled and handled however with the integration of Distributed Energy Resources (DER) and Grid-forming inverters, the grid today and grid of the future can no longer be defined with those few sets of equations. We now need technologies capable of identifying patterns on their own to provide give better insights. In this respect, AI stands out as the most promising technology.
- Transfer learning: The AI, specifically DL, also offers the advantage of applying the learning (pre-trained models) to improve generalization on other related tasks. This capability allows handling complex tasks with limited data by first training on similar problems with abundant data and then fine-tuning on the required one. Image processing and natural language processing are the most widely used applications of Transfer learning.
Challenges:
There are several challenges:
- Transparency, explain-ability and biases: In general, AI models lack transparency, which is critical for building confidence and trust in the explainability of reproducing the outcome or behavior under given grid conditions. Since AI makes decisions as a black-box model entirely based on data provided, if the data itself is sampled incorrectly i.e., sampled with some bias, then the decision will also not be unbiased. Addressing biased sampling has always been a challenge for AI experts, as it leads to the misrepresentation of correlation as the causation of problem as it is not easy to debug the model algorithms.
- Cyber risks of AI: Hallucination or extrapolation in making decisions or predictions may cause unintentional failure when there is a mismatch in trained data and different grid data. AI can be used to exploit weaknesses in the system, causing sophisticated attacks. To address this, AI may require continuous updates of AI algorithms, which is difficult in a substation operation network that doesn’t offer the required bandwidth.
- Cost: Cost-benefit analysis becomes one of the most important factors to realize if AI is feasible for the given application. The cost of training and maintaining AI based on application needs, should be weighed against the returns it can provide. If the overall cost outweighs the benefits, opting for human or less complex automation may be advantageous.
Trustworthy & Responsible Artificial Intelligence (AI)
Trustworthy AI, a term defined by the European Commission and based on ethics guidelines, refers to a standardized methodology for using technology. It outlines three main criteria for trustworthy AI:
- Lawful: respects all applicable laws and regulations
- Ethical: respects ethical principles and values
- Robust: systems need to be resilient, safe, and secure from a technical standpoint while considering the social environment
The technology needs to be lawful; it needs to be human-centric, and it needs to be technically sound as it considers the specific use case of the industry.
While above criteria are necessary, they may not be sufficient to ensure trustworthy AI, and human involvement may be required to align with social expectations.
In addition to being trustworthy, Responsible AI adds a layer of accountability, by requiring a value-driven approach , throughout the planning and deployment stages to ensure it never harms humans and the planet. The incorrect decision-making problem, due to biased sampling, discussed in the previous section, can be handled with Responsible AI. If law, ethics and values are considered during data collection process as well, and there is now AI act. The onus of Responsible AI lies with everyone developing and using AI technologies.
Evolving Grid AI-enabled Analytics
Power grid networks and equipment infrastructure are monitored and controlled by edge devices with sensors and actuators with some level of intelligent analytics. As illustrated in Figure 3, the evolution of grid edge analytics innovations progresses from a reactive management strategy that responds to failures after they occur to a more autonomous future state with self-healing capabilities.
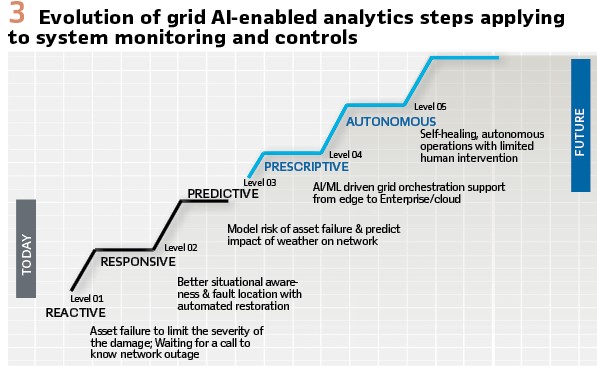
The majority of existing grid edge analytics are reactive to failures to limit damage, with limited responsiveness to restore automatically. The predictive stage may utilize system or asset models with a combination of physics and machine learning methods, including anomaly detection, classifications, and/or learned patterns. Further grid innovations can be applied to suggest potential implications and recommended actions. Finally, the autonomous grid with self-healing capabilities? would use edge-to-edge communications with very limited or no human intervention.
Predictive Transformer Health
Power transformers are among the most critical assets of a substation to maintain reliable and 24/7 power supply. With a typical age of 40-50 years, today more than 50% of world’s transformers are approximately 40 years old and are susceptible to failure. Electrical-related abnormalities and insulation aging, accounting for 52% of failure cases, highlight the importance of extending the lifespan of these assets, which is the primary goal of all utilities today. Traditionally, a transformer protection relay safeguards transformers from all electrical-related faults except for out of zone or through faults, while monitoring all electrical/thermal data. Additionally, Dissolved Gas Analysis (DGA) devices connected to a transformer oil outlet pipe collect and process oil samples (chemical analysis) to detect dissolved gases indicative of the transformer’s health condition. Historically, these two distinct systems-the electrical protection relay and DGA chemical analysis-have not been interconnected or analyzed together. However, since both systems provide much-needed asset health and performance management data, bringing them together offers a more comprehensive view of the transformer’s overall health as shown in Figure 4. This integrated approach, combining two systems (one protection relay with electrical/thermal functions, and a second DGA with chemical analysis) enables tracking of trending incipient fault events with the same timestamps, correlation between the electrical, thermal, and chemical models, and integrated asset health analysis and reporting.
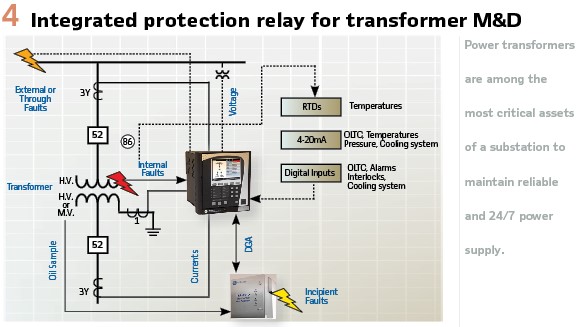
AI based Data correlation models: The models in a protection relay provide the ability to correlate electrical, chemical, and thermal data, facilitating the creation of individual trending models. Such models help identify correlations between different yet inter-related sets of data, such as the record of transformer loads compared to gas concentration data. A correlation model will help spot, for example, that a gas concentration is occurring every time a transformer is loaded in a particular way or tap changer is operated. This model can then be used to track transformer load, temperature, and gas concentration changes to uncover relationships between different measures. Similarly, various correlation models can be developed, combining physics-linked electrical, thermal, and chemical relevant time-series data. Each model provides unique insights on condition, performance or health, empowering operators to proactively safeguard transformer assets, and extending the time before a shut-down for maintenance or required decommissioning.
Integrated electrical, thermal and chemical analysis can be further used to design and develop AI/ML based correlation models. These models aim to understand the degree of correlation between relevant parameters and predict the trend of gases, for instance in correlation with temperature and load) , enabling the early forecast of a specific fault type occurrence
Capturing baseline data during a healthy state, deriving statistics, and then utilizing this baseline data to design an AI/ML model to calculate dynamic fault thresholds can help in accurately assessing the transformer’s condition with respect to its own baseline condition, rather than relying on static or fixed thresholds.
Furthermore, recommendations from these AI/ML-based models combined with a transformer risk management framework, can assist utilities in performing,
1) Risk assessment of the transformer population in service, especially critical transformers, and important connections
2) Condition assessment of individual transformers, identifying those which may require follow-up actions such as visual inspection, diagnostic measurements, and maintenance
3) Life cycle decisions for the transformer: determining if retirement, refurbishment, replacement, or relocation is necessary
Prescriptive Rotating Machines Applications
Rotating machines are among the most important components of any industrial plant, ensuring productivity along with reliable and safe motor operation. Traditionally, although motor protection relays may witness the evolution of a machine failure, no action is taken, as the primary role of the relay is to react (in terms of an alarm or trip) upon detection of the fault.
To achieve prescriptive motor asset monitoring, electric machine failure detection functions can be added, as show in Figure 5, differentiate among electrical, thermal and mechanical related abnormalities for the prognostics.
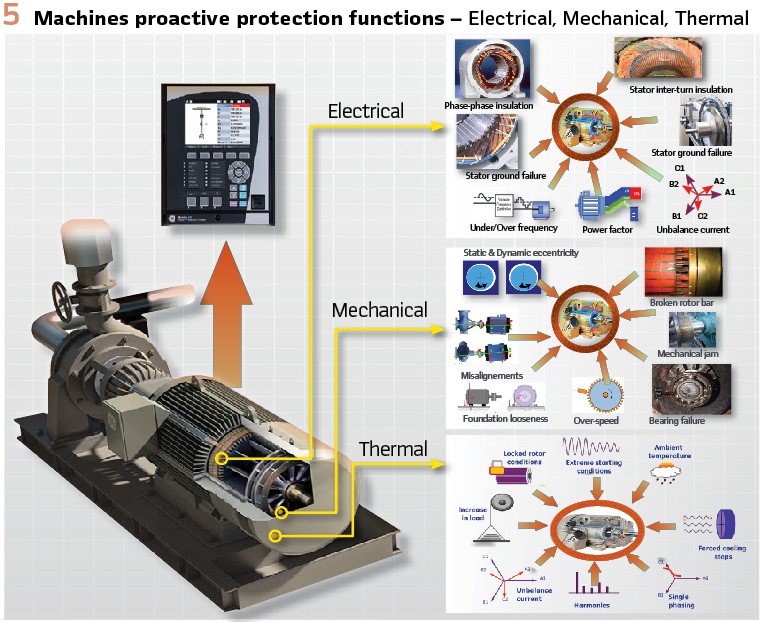
Prescriptive model functions for many electrical and mechanical abnormalities can be achieved using Electrical Signature Analysis (ESA), also known as Motor Current Signature Analysis (MCSA).
ESA is based on sensing stator current components at specific frequencies that are direct by-products of unique rotating air-gap flux components caused by faults such as bearing anomalies, static or dynamic eccentricity, shaft misalignments, foundation looseness, and broken rotor bars using advanced Fast Fourier Transform (FFT). Protection relay can capture ESA plots as illustrated in Figure 6.
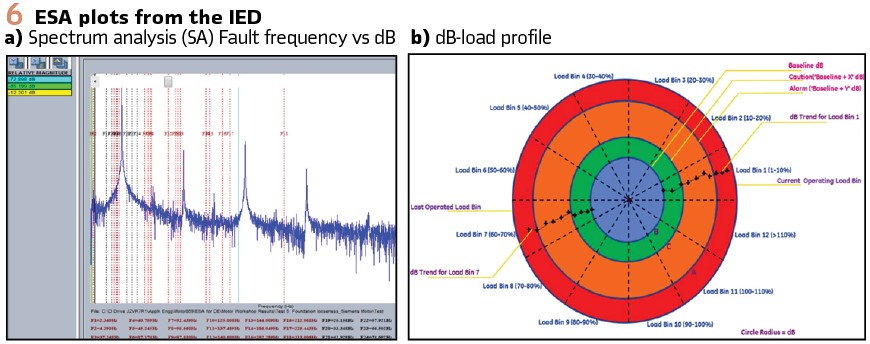
In Figure 6 (-a), the first plot, provides spectrum (FFT) analysis results of magnitudes (in dB) across the entire frequency spectrum. Thee red-dotted lines indicate the frequencies of interest for monitoring failure modes. The circular dB-load profile represents various peak dB level evolutions over different load bins to compensate for the loading effect.
The root cause of most stator failures is thermal stress, resulting in electrical stress. Overloading of a machine increases thermal stress on the machine, and hence the winding insulation, especially between the turns, degrades. This degradation causes stator inter-turn failures, eventually leading to ground or phase faults. Advancements in Machine Thermal Models to proactively monitor Thermal Capacity Used (TCU) in correlation with presented parameters is used to provide early warnings for preventive maintenance due to thermal stress.
The proactive data measurements of electrical, mechanical, and thermal Monitoring & Diagnostics (M&D) functions can be correlated and analyzed using AI/ML models to develop a digital twin of the motor asset. This digital twin depicts the failure conditions specific to each motor, including sub-types, based on historical data analysis combined with computed dynamic thresholds of fault indicating parameters.
Autonomous physics-informed Digital Twin of a Substation: One element of autonomous Digital Twin technology, primarily used within the power industry for detection purposes related to cybersecurity and troubleshooting of the grid.
The real-time autonomous technology enabling localization, forecasting, detection, and neutralization – helping to facilitate reliable and safer operation of the grid substation.
Many critical infrastructures, such as electric power grids, are operated via central control systems, acting as the brain of the network through data collection from sensors. Given the critical nature of these assets, protection and safety of these assets are imperative. Physics-informed technology is capable of not only detecting anomalies in the grid system but also localizing the anomaly and neutralizing their impact. With the modernization and digitalization of the grid, AI has the power (pun intended) to make great strides and address modern network challenges.
The major aspects of this technology relevant to substation are:
- Transformer Analytics AI Model in edge device helping in dynamic thresholding of alarms, predictive asset management, and event based (through fault) analytics
- Physics-informed AI Model for circuit-breaker and transformer control applications, addressing anomalies and cybersecurity intrusion issues at the OT layer
Conclusions
There is no question that grid innovations and digitalization are accelerating the decarbonization and electrification. Globally, the power grid industry needs to adopt new technologies, such as AI-based substation intelligence, while complying with AI regulations, to improve grid reliability, safety, sustainability, and affordability.
By default, artificial intelligence will not be human-centric if we are not ahead of it. Critical considerations and the role of AI in substations cannot be ignored – it is imminent. The question then turns to: how do we develop it responsibly? It’s clear that this is a powerful technology, so we need to stay ahead of the game and innovate with equal parts caution and optimism.
Trustworthy and responsible AI can enable and propel the energy transition into unchartered territory. There are several challenges to deploying AI that can be addressed with appropriate measures and regulations. Over the last decade, many companies have increased their adoption of AI technology and related investments. It’s clear that artificial intelligence is here to stay – within our industry and beyond.
Biographies:

Mital Kanabar is senior director of innovations with GE Grid Solutions – Grid Automation, Canada and adjunct Professor at the University of Ontario Institute of Technology (UOIT), Canada. He has a Ph.D. from the University of Western Ontario, M.Tech. Mital has 8 years of industrial R&D experience. He is currently vice-chairing the IEEE PES PSRC WG C19 on PDC standard; and WG K15 on centralized substation Protection and Control. He is an active member/contributor of many technical WGs at IEEE PSRC, CIGRE, NASPI, and IEC. He holds 8 patents and is the author of 40 papers and 3 magazine articles.

Sudhanshu Mishra is Engineering Manager – RTC Embedded SW Development with GE Grid Solutions – Grid Automation, India, having overall 17 years of experience. He leads team of engineers working on Real-Time controller firmware development, Customer application engineering and AI/ ML based solutions for grid applications. He holds master’s degree in Electronics & Telecommunications and an advanced certification in “Artificial Intelligence and Machine Learning” from IIIT Hyderabad, India. He has 4 patents on his name.

Dr. Balakrishna Pamulaparthy, PhD is currently working as Senior Engineer – Emerging Technologies at Office of Innovation, GENERAL ELECTRIC (GE), Hyderabad Technology Center, India in Smart Grid domain. He has overall 15+ years of Industrial R&D experience at GE. His research interests include Smart Grid, Substation/Distribution Automation, Grid Monitoring & Data analytics, Transformer/Motor Asset Management, DMS, SCADA and AMI.

Claudia Cosoreanu PEng., MBA is Grid Automation Chief Technology Officer and Protection, Automation, and Control Product Line Leader, responsible for Grid Automation product strategy and development globally. She has 25 years’ experience in power and utilities industry with expertise in product management, product development, advanced technologies, strategy, and system thinking. Claudia holds a Master of Science in Mechanical Engineering, Automation & Control and a Master of Business Administration in Marketing. She is the recipient of 2009 GE Women in Technology Award and 2015 GE Corporate Technology Staff Leadership Award. He holds three patents with application in the energy industry.