by ChatGPT and DALL-E, USA
Artificial intelligence (AI) is revolutionizing the field of electric power systems by enhancing protection and control mechanisms. These advancements are pivotal as they contribute to the stability, efficiency, and reliability of power grids, which are fundamental to modern societies. AI technologies, such as machine learning and deep learning, are being deployed to predict and manage complex scenarios in real time, addressing challenges that traditional systems might not handle efficiently. For example, AI can predict equipment failures before they occur, minimizing downtime and preventing large-scale disruptions. Additionally, AI facilitates the integration of renewable energy sources into the grid by predicting fluctuations in power supply and optimizing energy distribution.
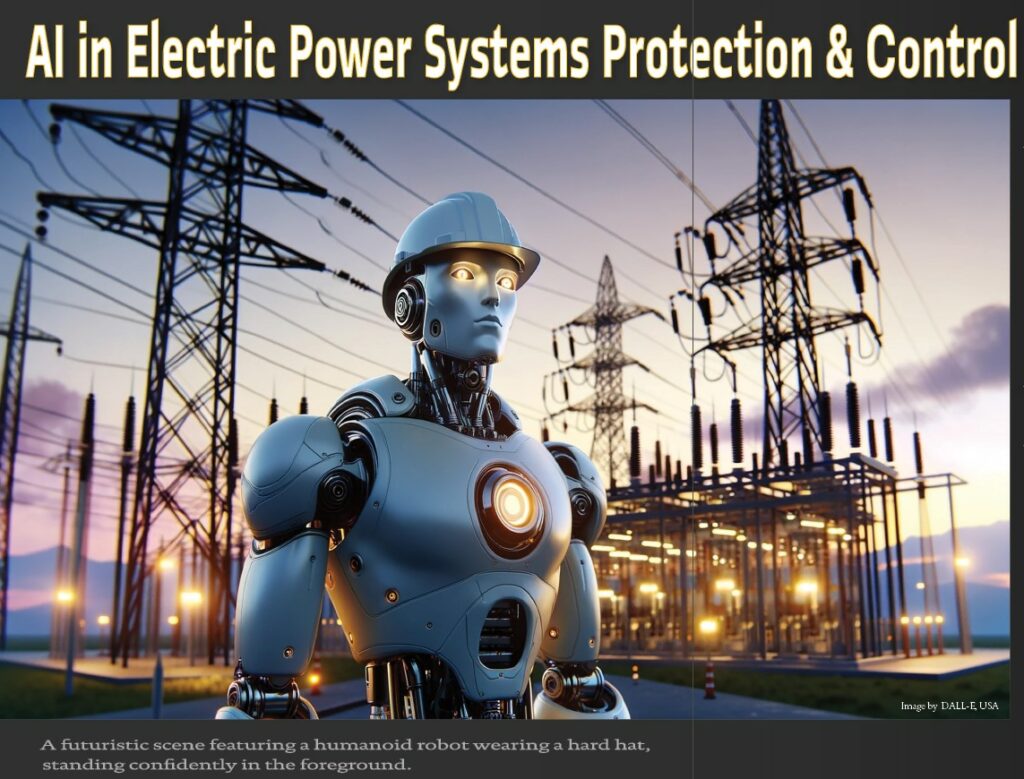
This not only ensures a steadier power supply but also enhances the sustainability of the power grid. Furthermore, AI algorithms improve the accuracy of fault detection and isolation, speeding up the restoration process after outages. This article explores the multifaceted applications of AI in the protection and control of electrical power systems, highlighting recent innovations, real-world implementations, and the future prospects of AI technologies in transforming electrical grids.
Early Concepts and Theoretical Foundations of AI The journey of artificial intelligence (AI) from the realm of mythology to a pivotal field of modern technology illustrates a fascinating transformation of an ancient dream into a contemporary reality. This transformation has roots stretching back to ancient myths and spans up to the sophisticated algorithms and theories that underpin today’s AI technologies.
Early Myths and Automata: The origins of AI can be traced back to the myths and stories of various ancient cultures, where automata (mechanical beings) and crafted life forms were often featured. In Greek mythology, for instance, Hephaestus, the god of craftsmanship, created mechanical servants made of gold, which could move and act on their own. Similarly, in Jewish folklore, the Golem—a clay figure animated by mystical means—reflects the idea of imbuing inanimate matter with life, an early conceptualization of artificial life.
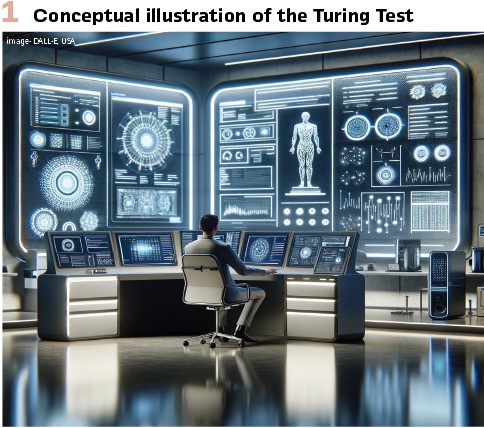
These mythological stories, while metaphorical, underline a long-standing human fascination with creating life-like entities, which laid the foundational curiosity and ambition necessary for the development of AI. As civilization moved into the Renaissance and the Age of Enlightenment, this fascination evolved into practical experimentation with mechanical automata. Notable examples include the mechanical knight by Leonardo da Vinci, designed in the late 15th century, and the various clockwork automata of the 18th century, which could perform complex tasks such as writing or playing music.
Theoretical Foundations and Formal Theories: The scientific groundwork for AI began with the formalization of logic and mathematics. Pioneers like Gottfried Wilhelm Leibniz, who conceived of a universal language or “calculus of thought” that would allow all conceptualizations to be processed like mathematical statements, contributed to early theoretical frameworks. In the 19th century, George Boole developed Boolean algebra, which would later be fundamental in computer logic and programming.
The concept of machines being capable of performing tasks reserved for human intellect was formally proposed by Alan Turing, a British mathematician, in his seminal 1950 paper “Computing Machinery and Intelligence.” Turing introduced the concept of what is now known as the Turing Test—a criterion of intelligence in a machine being its ability to exhibit indistinguishable behavior from that of a human. Turing’s work was preceded by the development of cybernetics by Norbert Wiener, which studied regulatory and control systems, and was analogous to the behavior of machines and living organisms.
Early Computers and Development of AI as a Discipline: The invention of the electronic computer during the Second World War was a leap forward in the development of AI. The ENIAC, built between 1943 and 1945, was one of the earliest examples. These machines, initially designed for ballistic calculations, soon showed the potential for much broader applications.
The formal establishment of AI as a distinct field occurred at a workshop held at Dartmouth College in 1956, organized by John McCarthy, Marvin Minsky, Nathaniel Rochester, and Claude Shannon. Here, the term “artificial intelligence” was coined, and the participants proposed that “every aspect of learning or any other feature of intelligence can in principle be so precisely described that a machine can be made to simulate it.”
Symbolic AI and Beyond: Following the Dartmouth workshop, the field of AI branched into several directions. One significant approach was symbolic AI, which focuses on manipulating symbols and using them to represent problems and knowledge in a manner that computers can use to solve complex tasks. The development of Lisp in 1958, a programming language suited for AI because of its excellent handling of symbolic information, was a significant milestone.
The progress in AI during this period included the creation of expert systems, which attempted to codify the knowledge and decision-making processes of human experts into a computer program. One of the most famous examples was MYCIN in the early 1970s, an expert system used in medical diagnosis to identify bacteria causing severe infections and recommend antibiotics.
The AI era of Machine Learning and Neural Networks
The field of artificial intelligence (AI) has undergone profound transformation over the last few decades, marked significantly by the shift from rule-based systems to machine learning models and the rapid evolution of neural networks. This new era is characterized by systems and models that learn from data, improve with experience, and perform tasks that were once deemed possible only for human intelligence.
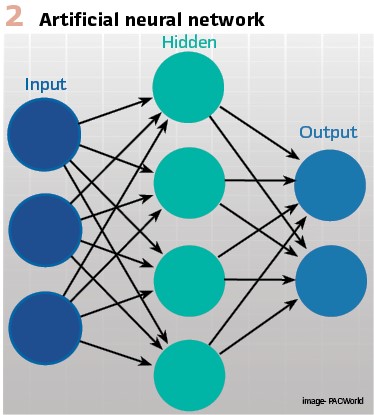
From Rule-Based Systems to Machine Learning: Rule-based AI systems, or expert systems, were the mainstay of AI from the 1970s through the late 1980s. These systems operated on a series of hardcoded rules and logic that defined how they should behave in response to specific inputs. They were designed to mimic the decision-making ability of human experts by following an extensive set of if-then rules derived from the domain’s knowledge. While effective within their limited scope, rule-based systems were inherently inflexible, unable to learn from new data, and labor-intensive to update as they required manual tweaking of rules.
The limitations of rule-based systems led to the advent of machine learning in the 1980s, a paradigm shift initiated by the need for more adaptive systems capable of generalizing from data patterns. Machine learning algorithms, driven by statistical methods, allow systems to learn from and make predictions or decisions based on data. This ability to learn and adapt without being explicitly programmed for specific tasks marked a significant advancement in AI capabilities.
Introduction and Evolution of Neural Networks: Neural networks, inspired by the biological neural networks that constitute animal brains, are a set of algorithms, modeled loosely after the human brain, that are designed to recognize patterns. They interpret sensory data through a kind of machine perception, labeling, or clustering raw input. The basic building blocks of these networks are neurons — nodes that are interconnected and pass signals to each other. These signals are processed through the network layers, which perform various transformations on the input data to learn to perform tasks without having explicit instructions.
Although the concept of neural networks dates back to the 1940s with the work of Warren McCulloch and Walter Pitts, significant advancements were stifled until the 1980s due to the lack of powerful computational resources and the understanding of key theoretical elements, such as how to effectively train large networks.
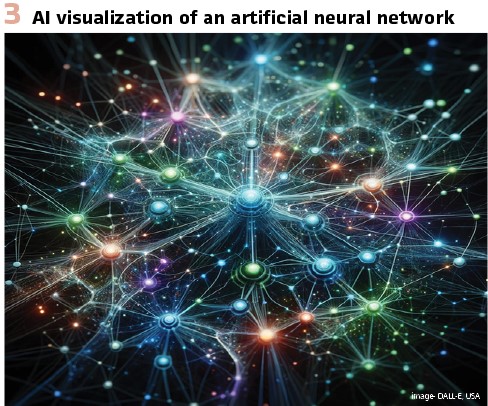
Rise of Deep Learning: The resurgence of interest in neural networks in the 21st century, especially with the advent of ‘deep learning’, has been transformative. Deep learning involves training large neural networks with many layers (hence “deep”) on vast amounts of data. These networks perform remarkably well in tasks such as image and speech recognition, language translation, and even complex decision making. The pivotal moment for deep learning came in 2012 when a model designed by Alex Krizhevsky, Ilya Sutskever, and Geoffrey Hinton significantly outperformed other models in the ImageNet competition, a benchmark in visual object recognition.
Deep learning has benefited greatly from the availability of large data sets and advances in computing hardware, such as GPUs (Graphical Processing Units) that can perform computations much faster than traditional CPUs for the tasks involved in training neural networks. This has allowed deep learning models to scale up dramatically, increasing both their complexity and their capability.
Reinforcement Learning: Another significant development in AI is reinforcement learning, an area of machine learning concerned with how software agents ought to take actions in an environment so as to maximize some notion of cumulative reward. Unlike supervised learning where the model is trained with the correct answer key, reinforcement learning operates through a process of trial and error, where the model learns to achieve a goal in an uncertain, potentially complex environment. This methodology has been famously applied in systems that learn to play-and master-complex games, such as AlphaGo developed by Google DeepMind.
Reinforcement learning models are particularly interesting because they can learn to make a sequence of decisions. The agent learns to achieve a goal in an uncertain, potentially complex environment. This is achieved through exploration (trying unfamiliar actions) and exploitation (leveraging known actions), balancing between these two modes to maximize the overall reward.
The Evolution of AI in the 21st Century
The 21st century has witnessed an unprecedented acceleration in the field of artificial intelligence (AI), fueled largely by significant advances in computational power, data availability, and the integration of technologies such as big data analytics, cloud computing, and the Internet of Things (IoT). This confluence has not only transformed AI from a largely theoretical discipline into one of the most practical and impactful fields in technology but has also reshaped industries, economies, and day-to-day human interactions.
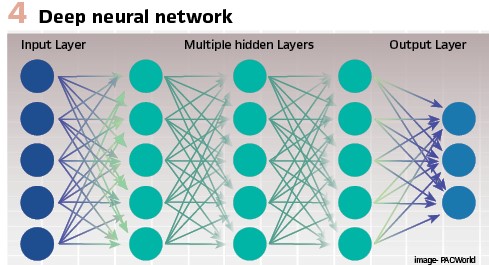
Advances in Computational Power & Data Availability: The explosion in AI capabilities can largely be traced back to the dramatic increase in computational power. Moore’s Law, which posited that the number of transistors on microchip doubles about every two years though the cost of computers is halved, has held true for much of the technology’s development, leading to faster, smaller, and more affordable processors. This trend has enabled the development of high-performance computers required for AI. The advent of GPUs (Graphics Processing Units) and TPUs (Tensor Processing Units) specifically designed for handling the massive computations necessary for AI tasks, especially those involving deep learning, has significantly decreased the time required for training models and increased the complexity of tasks that can be undertaken.
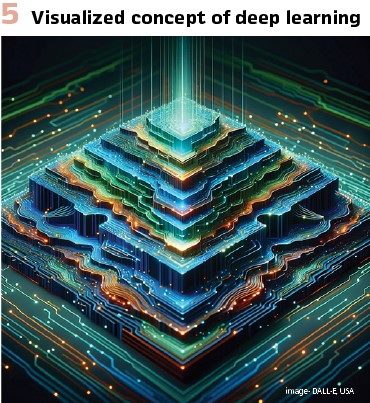
Parallel to the surge in processing power, there has been an exponential growth in data generation and collection—termed “Big Data”. This data comes from myriad sources: social media feeds, internet search queries, e-commerce transactions, and IoT devices, among others. The digital universe is expected to reach 44 zettabytes by 2020. AI thrives on large datasets; the more data an AI system can access, the better it can learn and the more accurate it becomes. Hence, the availability of big data has been a critical enabler in the development of more sophisticated AI models.
The Rise of Big Data Analytics: Big data analytics refers to the process of collecting, organizing, and analyzing large sets of data to discover patterns and other useful information. This field has grown in tandem with AI, as the insights gleaned from big data analytics are often fed into AI systems. Techniques such as predictive analytics use statistical algorithms and machine learning techniques to identify the likelihood of future outcomes based on historical data. This is invaluable for industries ranging from healthcare, where predictive models can forecast disease outbreaks, to retail, where they can predict consumer buying behaviors.
Impact of Cloud Computing: Cloud computing has been another crucial factor in the AI boom. By allowing data and AI systems to be hosted on the Internet, rather than on local computers or servers, cloud computing has dramatically lowered the barrier to entry for utilizing AI technologies. It provides businesses and individuals with access to powerful computing resources and vast amounts of storage space without the need for significant upfront investment in physical hardware. This democratization of access has allowed startups and smaller companies to deploy AI solutions that were previously the preserve of large corporations.
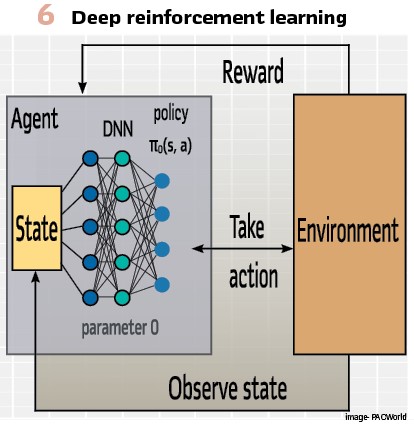
Moreover, cloud platforms often offer as-a-service options for machine learning and deep learning, which further simplifies the process of developing and deploying AI models. These platforms continuously update and maintain their hardware and software, ensuring that users always have access to the latest advancements without additional cost or effort on their part.
The Role of IoT in AI’s Growth: The Internet of Things (IoT) describes the network of physical objects-“things”-that are embedded with sensors, software, and other technologies for the purpose of connecting and exchanging data with other devices and systems over the Internet. IoT has exponentially increased the number of data points available for analysis, providing AI systems with a more comprehensive view of the world.
IoT devices permeate various sectors, including healthcare (with devices that monitor patient health in real time), transportation (with smart sensors for fleet management and vehicle safety), and manufacturing (with sensors to monitor and optimize industrial processes). The data collected from these devices not only helps in immediate operational functions but also feeds into larger AI-driven analytics, improving system efficiencies and capabilities over time.
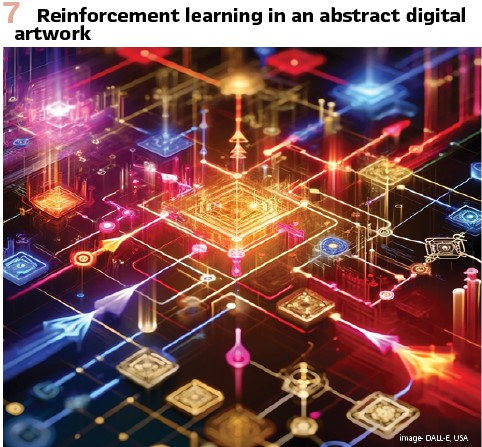
The Integration of AI into Electric Power Systems
The integration of Artificial Intelligence (AI) into electric power systems represents a transformative shift towards more efficient, reliable, and intelligent grid management. By harnessing the capabilities of AI, particularly machine learning (ML) and deep learning, utilities are now better equipped to predict and identify system faults, enhance real-time monitoring, optimize power flow, and stabilize the grid. This integration not only promises significant improvements in operational performance but also in reducing downtime and maintenance costs through predictive maintenance strategies.
AI for Fault Detection and Diagnosis: Traditionally, fault detection in electric power systems has relied on rule-based systems that require extensive manual inputs and adjustments. These methods can be slow and often lack the ability to adapt to new or unseen fault conditions, leading to delays in response times and potential escalations in system failures. In contrast, AI-driven approaches, especially those utilizing machine learning models, offer a dynamic solution capable of learning from vast datasets, including historical performance data, real-time sensor data, and environmental conditions.
Machine learning models can be trained to recognize patterns that precede faults or anomalies, enabling them to predict and identify issues much faster and with greater accuracy than traditional methods. For example, a deep learning model can continuously analyze the electrical load data and instantly detect deviations that may indicate a fault or an emerging issue, thereby prompting preemptive actions to mitigate potential impacts.
Real-Time Monitoring and Anomaly Detection: AI applications extend significantly into real-time monitoring and anomaly detection. One such application is the use of neural networks to monitor power lines and substations continuously. These networks are trained on historical data, including instances of equipment failures, weather conditions, and load demands, allowing them to detect anomalies that could suggest equipment malfunctions or external threats like physical damages or cyber-attacks.
For instance, AI-driven systems in California are currently used to analyze weather data, predict potential fault lines during wildfires, and dynamically reroute power to minimize fire risk and ensure supply continuity. Similarly, in Europe, grid operators use machine learning to detect and respond to anomalies in real-time, enhancing their ability to maintain system stability even under fluctuating conditions and unexpected load variations.
Adaptive Control Systems for Power Flow and Voltage Regulation: AI-powered adaptive control systems represent another significant advancement in the smart grid domain. These systems utilize algorithms to optimize power distribution based on real-time data, thereby enhancing efficiency and reliability. For instance, reinforcement learning, a type of machine learning where algorithms learn to make specific decisions by trying to maximize a reward signal, can be used to adjust power flows automatically and manage voltage levels across the grid.
This capability is crucial for integrating renewable energy sources, such as solar and wind, which can introduce variability in power generation. AI systems can dynamically adjust to changes in power supply and demand, ensuring optimal grid performance and preventing voltage instability or outages.
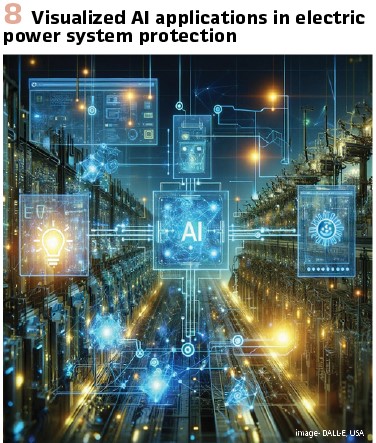
AI in Demand Response and Grid Stabilization: Demand response programs are critical for grid stability, especially as the penetration of renewable energy sources increases. AI can significantly enhance these programs by predicting peak load periods and enabling utilities to manage demand more effectively. Machine learning models analyze consumption patterns, weather forecasts, and economic factors to forecast demand peaks and suggest optimal responses such as temporary price adjustments or automatic reduction of consumption in commercial and residential buildings.
Moreover, AI applications in grid stabilization include deploying algorithms that can instantly calculate and execute the best response to sudden changes, such as a renewable source suddenly going offline. These algorithms not only balance the load to prevent a power failure but also ensure that the transition is smooth and largely unnoticed by consumers.
Implementations of AI in power system protection
The integration of Artificial Intelligence (AI) into power system protection has revolutionized the way electricity grids are monitored, analyzed, and maintained. AI’s influence spans several critical areas, including fault detection, predictive maintenance, and real-time operational adjustments. This deployment not only enhances the efficiency and reliability of power systems but also addresses complex challenges that traditional methods struggled with.
AI in Fault Detection and Diagnosis: One of the most impactful applications of AI in power system protection is in the area of fault detection and diagnosis. Traditional systems rely on predefined settings and simple algorithms that often fail to cope with the dynamic nature of modern power grids, which include renewable energy sources and variable load patterns. AI models, particularly those based on machine learning and deep learning, excel in these environments due to their ability to learn from vast amounts of data and adapt to new, previously unseen scenarios.
For example, utilities are now using AI to monitor transmission lines in real time. By analyzing data from sensors and weather stations along with historical fault data, AI systems can predict and identify potential issues before they lead to failures. These systems can differentiate between normal fluctuations and those that signify problems such as a damaged cable or an overloaded circuit. This proactive approach not only prevents outages but also minimizes the wear and tear on infrastructure by avoiding unnecessary stress on components.
Predictive Maintenance: Predictive maintenance is another area where AI significantly contributes to power system protection. Traditional maintenance schedules are typically based on estimated life expectancies and periodic checks, which often lead to either premature maintenance or unexpected failures. AI changes this scenario by enabling a condition-based maintenance strategy.
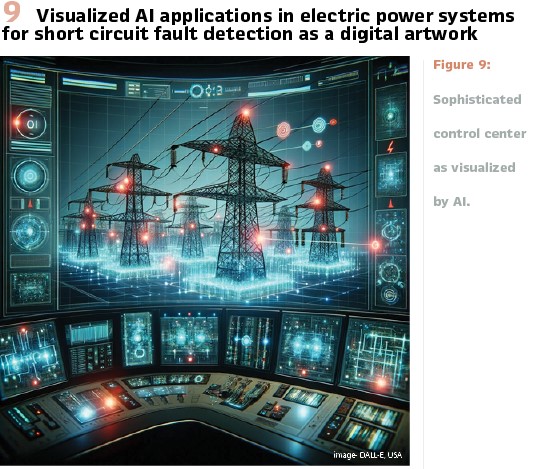
Using data collected from sensors installed on various components like transformers, breakers, and bushings, AI algorithms analyze the operating conditions and performance to predict when maintenance should be performed. This method ensures that maintenance is only conducted, when necessary, which optimizes resource use and reduces downtime. For instance, a North American energy provider implemented AI-driven predictive maintenance and reported a reduction in unexpected equipment failures by 30%, leading to increased grid reliability and reduced operational costs.
Real-Time System Adjustments: AI also plays a crucial role in real-time system adjustments in power grids. Dynamic line rating systems, which use real-time weather data to calculate the maximum safe operational capacity of power lines, are an example. AI algorithms analyze temperature, wind speed, and solar irradiation to adjust the power flow, which can prevent overheating and reduce the risk of line sagging. Such systems allow for more power to be transmitted safely during favorable conditions, thus optimizing the usage of existing infrastructure.
Challenges in Implementation: While the benefits of AI in power system protection are clear, the implementation of these technologies is not without challenges. The accuracy of AI models heavily depends on the quantity and quality of the data they are trained on. In many cases, collecting comprehensive and clean data from power systems can be difficult, especially from older components that were not designed with data collection in mind.
Additionally, the integration of AI into existing power systems often requires significant upfront investment in both technology and training. Power system operators need to be trained not only on how to use the new systems but also on how to trust and interpret the decisions made by AI, which can sometimes seem opaque or counterintuitive.
Cybersecurity is another critical concern. As power systems become more connected and smarter, they also become more vulnerable to cyber-attacks. Ensuring the security of AI-driven systems is paramount to protect critical infrastructure from potential threats.
Biographies:
ChatGPT is an AI language model developed by OpenAI, based on the GPT (Generative Pre-trained Transformer) architecture. Launched in November 2022, ChatGPT is designed to generate human-like text responses in a conversational manner. It is trained on a diverse dataset from books, websites, and other texts to perform tasks like answering questions, providing explanations, and generating creative content. ChatGPT aims to assist users across various domains, including education, customer support, and content creation, by offering insights, solving problems, and engaging in detailed discussions. Its capabilities are continuously evolving, driven by advances in AI research and user feedback.
DALL-E is an AI program developed by OpenAI, first introduced in January 2021. Named as a portmanteau of the famous surrealist artist Salvador Dalí and Pixar’s animated character WALL-E, it utilizes a version of the GPT-3 architecture to generate images from textual descriptions. This AI is capable of creating original, high-quality images and art from a simple text prompt, showcasing the ability to understand and manipulate concepts across different styles and contexts. DALL-E pushes the boundaries of creative AI, demonstrating its potential in assisting and augmenting human creativity in fields such as graphic design, advertising, and digital art.